3 Types of Data to Fuel the Fire
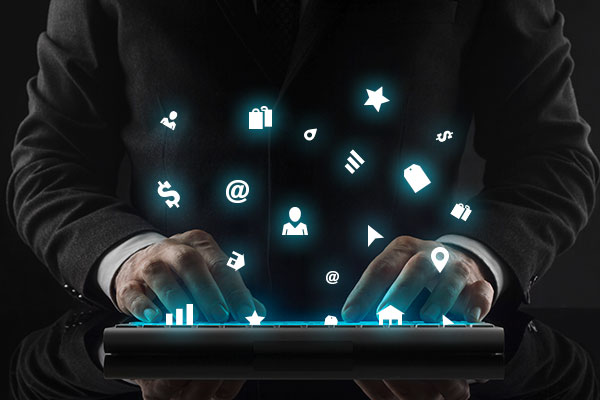
Article Highlights:
- How combining three types of data helps make more informed decisions.
- Leads with more data close 4.1x as often.
Predictive data is a hot topic. Companies like Target and Amazon are leading the charge to analyze customer behaviors and profiles to determine buying likelihood. What do they have in common? The fuel to their fire.
Solid predictive analytic engines are fueled by three types of data: demographic, behavioral, and transactional. They’re hot on their own, but when combined together, the results are like wildfire. With a single data point, you might know who is going to buy. If you add another, you’ll know what exactly they’ll buy. You’ll go from scoring customers here and there, to disrupting the buying cycle and retaining customers 4.1x as often[1].
Here’s a breakdown of what goes into each type of data and how they fuel predictive analytics engines:
- Demographic
This is defined as statistical information about a population, based on DMS or census data. These demographics create profiles based on characteristics such as age, gender, location, job, income, education, housing, and more.
- Behavioral
This makes up any action a customer has taken outside your dealership’s four walls, including vehicle title transfers and change of addresses.
- Transactional
This includes anything that has occurred inside your dealership. Buying or leasing a vehicle (and what the price was on the deal), financing rates, amount left on the loan, warranty information, and amount of service visits in a given period are all examples of transactions in the DMS or CRM.
Fueling the Fire
Customers behave like those they are around, so profile segmentation is present across all three. To make appropriate predictions on what a customer will do next, the engine groups customers into different profiles based on their demographics and similar customers. From there, it will analyze behavioral and transactional tendencies to leverage past decisions and predict what a customer will do next.
Here’s an example: Your customer moved to a new neighborhood. Everyone in this neighborhood drives a high-end SUV. Not only does this customer want to purchase a high-end SUV because everyone around her drives one, but she’s also likely to make the upgrade because of her move. This new neighborhood indicates a higher average salary, which leads to higher payment options.
Another example: A customer’s warranty has just expired. He entered outside the mileage parameters, so it’s the prime time to consider options. Or perhaps the customer buys the latest model every year, suggesting he is keen on maintaining a “premier” status. These factors are a major leg up for approaching a customer about upgrading their vehicle.
Thinking Forward
Your predictive analytics engine should be comprised of not one, not two, but three different types of data. Demographic, behavioral, and transactional data help make more informed predictions by leveraging the unique data points from one another to determine who is going to buy, what they’re going to buy, and when they’re going to buy it.
[1] Reynolds study
Related Articles:
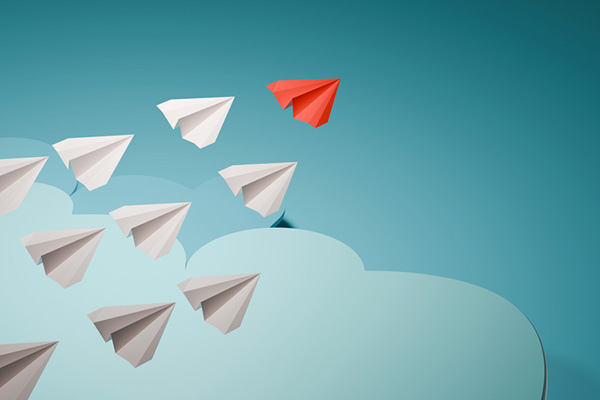
Leading The Way: 3 Tips to Help Your Team Find Quality Prospects
Customers have unlimited options in today’s market, so it’s important your team makes the most of every interaction. Failure to do so jeopardizes long-term customer…
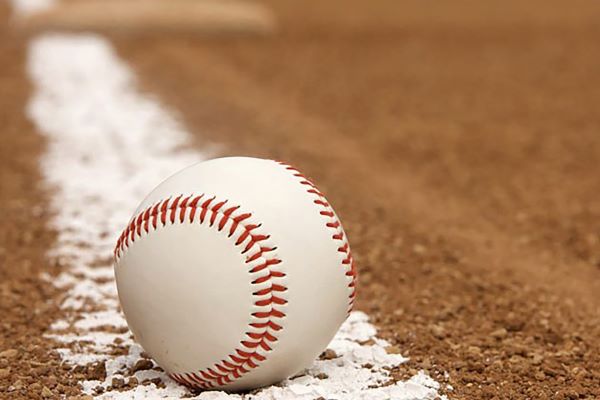
Three Things Baseball Can Remind Us About Funding a Deal
As we head into summer, there are many things to look forward to, like children laughing in the park and ice cream trucks spreading familiar…
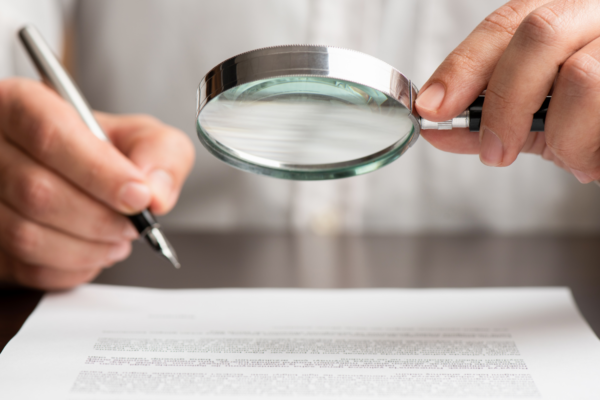
Understanding the FTC’s Enforcement Tools and How You Can Create Your…
The Federal Trade Commission (FTC) has intensified its focus on dealerships and is attempting to add new regulatory practices to their already robust arsenal. If…
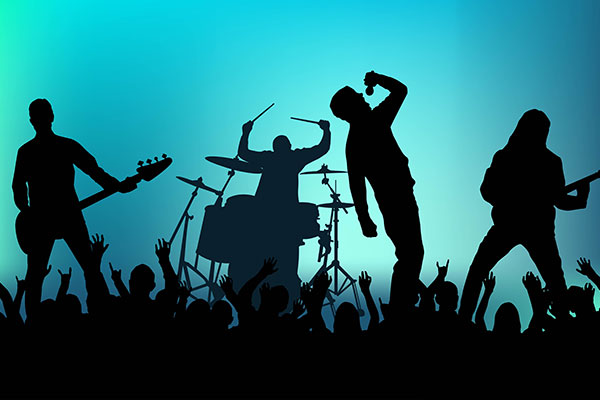
Is your sales team staying in tune?
Everybody enjoys a good musical performance. The singer producing an amazing melody on the microphone and the band backing them up with their instruments. The…